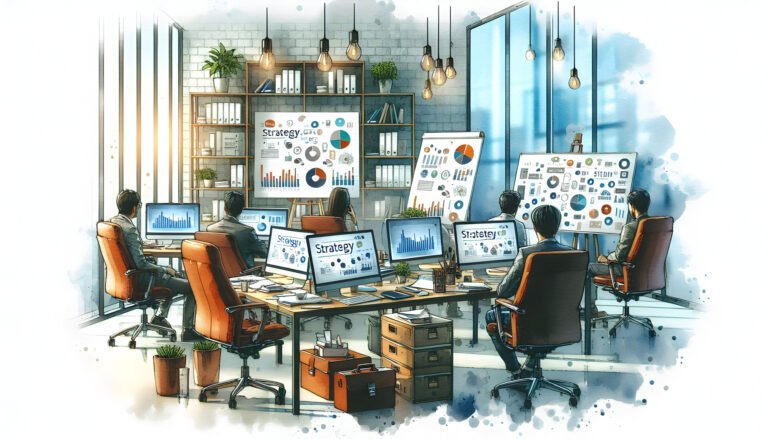
Generative AI tools have become more available and powerful than ever before. We need to understand the ethical side of using these tools. People who work with AI systems regularly must use these technologies responsibly and thoughtfully. AI capabilities are advancing fast, bringing amazing opportunities and vital ethical challenges we need to tackle.
This piece takes you through everything in AI ethics. We’ll get into privacy concerns, bias implications, and transparency challenges. These factors affect how we use AI systems daily. You’ll learn practical ways to ensure ethical usage. The topics range from data protection to environmental effects. This knowledge will help you make better decisions about generative AI in both personal and professional settings.
Privacy and data protection in generative AI represent one of the most important ethical challenges in modern technology. The European Union introduced the General Data Protection Regulation (GDPR) in 2018, which created stringent guidelines for handling personal data and set a global standard for data protection 1.
Generative AI systems process personal data through multiple stages – from dataset creation to model deployment. The process becomes especially complex when you have personal data processing happening behind the scenes 2. At the time we get into the training data, web scraping techniques collect personal information without people’s knowledge or consent 2.
Several essential data protection principles require our attention:
Generative AI presents a unique challenge compared to conventional applications. These models lack a “delete” button, which makes removing specific data points impossible after their incorporation 3.
Data breaches pose severe risks to organisations. The United States has seen 13 states pass detailed data protection laws rapidly over the last three years 4. This risk becomes especially concerning with sensitive information that can leak into AI systems – from internal company projects to intellectual property and personal records 3.
Several technical measures have emerged to reduce these risks. Organisations now use data anonymization techniques and federated learning approaches. Each participant keeps their dataset locally and shares only model parameters 1. Note that anonymization doesn’t guarantee complete privacy, and the process brings its own set of challenges 5.
Ethical considerations in generative AI face a significant challenge related to bias and fairness. Research has found that AI systems learn bias patterns from their training data. These patterns create vital ethical implications 6.
Bias in AI emerges from multiple sources, with training data being the main culprit. A deep dive into training datasets reveals unbalanced representations that can distort AI outputs. To cite an instance, see how research has shown AI-generated images portray higher-paying professions like CEOs and lawyers mostly with lighter skin tones 7. The core team has identified several distinct types of bias in training data:
Bias in AI systems shows up clearly in real-life applications. A Bloomberg analysis found AI-generated images of high-paying jobs that mostly depicted males with lighter skin tones 8. Large language models in healthcare can make race-based medical decisions that put patient care at risk 8. A TELUS Digital survey revealed that 32% of people believe AI bias has caused them to miss opportunities 8.
Several effective approaches can tackle these challenges. Our research reveals that pre-processing techniques like balanced dataset creation and synthetic data generation can substantially reduce bias 9. The results look promising with:
Data-focused solutions:
Our team discovered that fairness metrics during model development help calculate and address bias better. The development of “Constitutional AI” systems shows great promise, as these systems come with built-in ethical principles that guide their outputs 8.
AI systems’ inner workings create complex challenges for ethical deployment. These sophisticated systems have become a big deal as it means that they surpass our knowing how to explain their decisions.
The most important challenge we face today stems from what experts call the “black box” phenomenon. Users can see inputs and outputs but cannot fully understand how AI systems work internally 10. The system’s opacity hides the specific steps and data transformations that lead to decisions. This becomes especially when you have to understand how and why these systems produce certain outcomes 10.
People increasingly worry about telling AI-generated content apart from human creations. The biggest challenges we face include:
Digital watermarking offers a promising solution. Algorithms can embed identifiers into content files without affecting their usability 11. These watermarks connect to online registries that contain significant information about AI tools, creation dates, and user involvement 11.
Model interpretability goes beyond technical requirements—it plays a significant role in building trust and ensuring responsible AI deployment. Recent studies show that only 40% of AI systems offer simple transparency details about their data size, sources, and curation processes 12. This transparency gap exists in training datasets, fine-tuning processes, and labelling procedures 12.
The emergence of Explainable AI (XAI) tools brings hope by giving an explanation of how generative models work internally 10. These tools make complex systems easier to understand without compromising their performance. We can identify how input prompts affect outputs through techniques like feature attribution, which helps detect potential biases and collateral damage in content generation 10.
Generative AI’s environmental footprint raises serious ethical concerns that we must tackle as technology evolves. AI operations now consume energy at unprecedented rates. Experts project that AI’s global electricity usage could surge to somewhere between 85.4 and 134 terawatt hours each year by 2027 13.
Generative AI operations demand significant resources. A single AI-powered search uses four to five times more energy than traditional web searches 14. These large AI systems could match the energy consumption of entire countries in the coming years 14. The cooling requirements present another challenge, especially when you have massive processors that need substantial amounts of fresh water both for cooling and electricity generation 14.
Research shows some remarkable facts about how AI affects our environment:
A single large AI model’s training releases about 502 tonnes of carbon dioxide into the atmosphere. This equals the yearly emissions from 112 cars running on gasoline 15
The world’s data centres contribute significantly to global warming. They produce 2.5% to 3.7% of worldwide greenhouse gas emissions 15
Each time someone uses ChatGPT, it adds 4.32g of CO2 to our atmosphere 15
New solutions in green AI development look promising. The BigScience project in France showed we can build models as large as GPT-3 with a much smaller carbon footprint 14. Several positive changes are happening in this space.
Companies now use energy-efficient algorithms and optimise AI infrastructure to minimise environmental effects 16. Techniques like pruning and quantization create leaner AI models that need less computing power 16. On top of that, more organisations invest in green data centres that run on renewable energy. This cuts down the carbon footprint of AI operations by a lot 16.
Life cycle analysis of AI systems helps us spot ways to improve development and deployment 16. These insights let us make smart choices about green AI practises without sacrificing performance.
Generative AI’s ethical considerations encompass significant aspects that define responsible technology usage. Privacy protection, bias mitigation, transparency requirements, and eco-friendly practises create interconnected challenges that just need attention. These challenges come with practical solutions. Reliable data protection measures, bias detection tools, explainable AI frameworks, and energy-efficient computing practises help address these issues. Organisations and people who welcome these ethical guidelines position themselves better for responsible AI adoption. They build trust and maintain compliance effectively.
Generative AI’s future relies on our steadfast dedication to ethical implementation and responsible development. We have a long way to go, but we can build on this progress in constitutional AI, eco-friendly computing, and transparent model development. This shows we know how to address ethical challenges. Each step toward ethical AI usage builds public trust and advances technological capabilities. Responsible breakthroughs and powerful AI capabilities work together harmoniously. This balanced approach will give generative AI the power to drive progress while protecting individual rights and environmental sustainability.
What ethical issues should be considered when utilising generative AI?
When using generative AI, it’s crucial to be vigilant about the potential for your data to be sold or shared with third parties for purposes such as marketing or surveillance. Always exercise caution when providing sensitive, confidential, or proprietary information.
Can you list some key ethical considerations for AI projects?
Certainly, the top ethical considerations for AI projects include fairness and bias, transparency, privacy, safety, explainability, human oversight, and data best practises.
What are the primary ethical considerations when employing generative AI technologies?
Key ethical considerations when using generative AI encompass data privacy, bias and fairness, accountability and transparency, robustness and security, as well as broader social and ethical implications. It’s also important to adhere to ethical guidelines and best practises.
What are some examples of ethical considerations in AI?
Ethical considerations in AI involve issues such as data responsibility and privacy, fairness, explainability, robustness, transparency, environmental sustainability, inclusion, moral agency, value alignment, accountability, trust, and the potential misuse of technology.
[1] – https://lamarr-institute.org/blog/ai-training-data-bias/
[2] – https://www.edps.europa.eu/system/files/2024-06/24-06-03_genai_orientations_en.pdf
[3] – https://stackoverflow.blog/2023/10/23/privacy-in-the-age-of-generative-ai/
[4] – https://iapp.org/news/a/data-protection-issues-for-employers-to-consider-when-using-generative-ai
[5] – https://www.alation.com/blog/data-ethics-in-ai-6-key-principles-for-responsible-machine-learning/
[6] – https://arxiv.org/pdf/2304.07683
[7] – https://www.lis.ac.uk/stories/how-ai-image-generators-make-bias-worse
[8] – https://www.telusdigital.com/insights/ai-data/article/mitigating-genai-bias
[9] – https://medium.com/@jam.canda/ethical-machine-learning-creating-fair-and-unbiased-models-24aac2b6345b
[10] – https://www.posos.co/blog-articles/explainable-ai-part-1-understanding-how-ai-makes-decisions
[11] – https://www.forbes.com/sites/billrosenblatt/2023/07/22/google-and-openai-plan-technology-to-track-ai-generated-content/
[12] – https://theodi.org/news-and-events/blog/ai-data-transparency-understanding-the-needs-and-current-state-of-play/
[13] – https://www.informationweek.com/sustainability/reducing-the-environmental-impact-of-artificial-intelligence
[14] – https://www.nature.com/articles/d41586-024-00478-x
[15] – https://planbe.eco/en/blog/ais-carbon-footprint-how-does-the-popularity-of-artificial-intelligence-affect-the-climate/
[16] – https://technologymagazine.com/articles/green-ai-building-sustainability-into-ai-initiatives
Join the AI by Riz community and get exclusive tips, expert insights, and the latest in generative AI delivered straight to your inbox. From practical AI hacks for everyday tasks to deep dives into industry-specific applications, our newsletter keeps you informed, empowered, and ready to lead with AI.
Unlock the power of generative AI to enhance productivity and growth across professional roles. From healthcare to finance, AI by Riz provides tailored learning paths, hands-on tutorials, and innovative insights to help you master AI and transform your career.
info@example.com
(123) 456 7890
121 King Street, NY, USA